The origins of cognitivism and human intelligence
The origins of cognitivism
THE cognitivism is an approach in psychology that focuses on the internal mechanisms of thought, treating the human mind as an information processing system. The origins of this approach date back mainly to the 1950s and 1960s, as a reaction to behaviorism, which was very dominant at the time and which focused exclusively on observable behaviors, neglecting internal mental processes.
THE foundations of cognitivism are based on the work of notable figures such as Jean Piaget, who studied cognitive development in children, or Noam Chomsky, whose critique of behaviorist psychology and theories of verbal learning was a pivotal for the orientation of psychology towards a more in-depth study of the mind and its capacities.
It is no coincidence that the emergence of cognitivism coincides with the rise of computer science and cybernetics, which have provided new models and metaphors for conceptualizing the operations of the mind. For example, information processing in memory, algorithmic operations, and the use of computational models to represent thought processes.
Human Intelligence
L’human intelligence is a complex faculty that encompasses diverse abilities such as problem solving, comprehension, learning, adaptation to new contexts, creativity, and manipulation of symbols and concepts. Within the cognitivist framework, human intelligence is often envisioned as the result of cognitive processes that can be broken down and analyzed scientifically.
One of the great advances in understanding human intelligence through the prism of cognitivism has been the development of cognitive psychology, a discipline dedicated to studying mental processes and their influence on human behavior. Neuroscience research has also contributed to our understanding of intelligence, uncovering the underlying functioning of the brain and exploring how brain structures participate in cognitive processes.
Theories of multiple intelligence, proposed by psychologists such as Howard Gardner, have also enriched the debate by suggesting that intelligence is not a single, general ability, but rather a set of specific and independent skills. .
Furthermore, current understanding of human intelligence is profoundly influenced by artificial intelligence (AI). Paradoxically, in seeking to create machines that mimic human intelligence, researchers have gained new insights into the nature of our own intelligence.
This back-and-forth between AI and cognitive psychology has led to improvements in both fields, leading to significant advances in our understanding and ability to model intellectual processes.
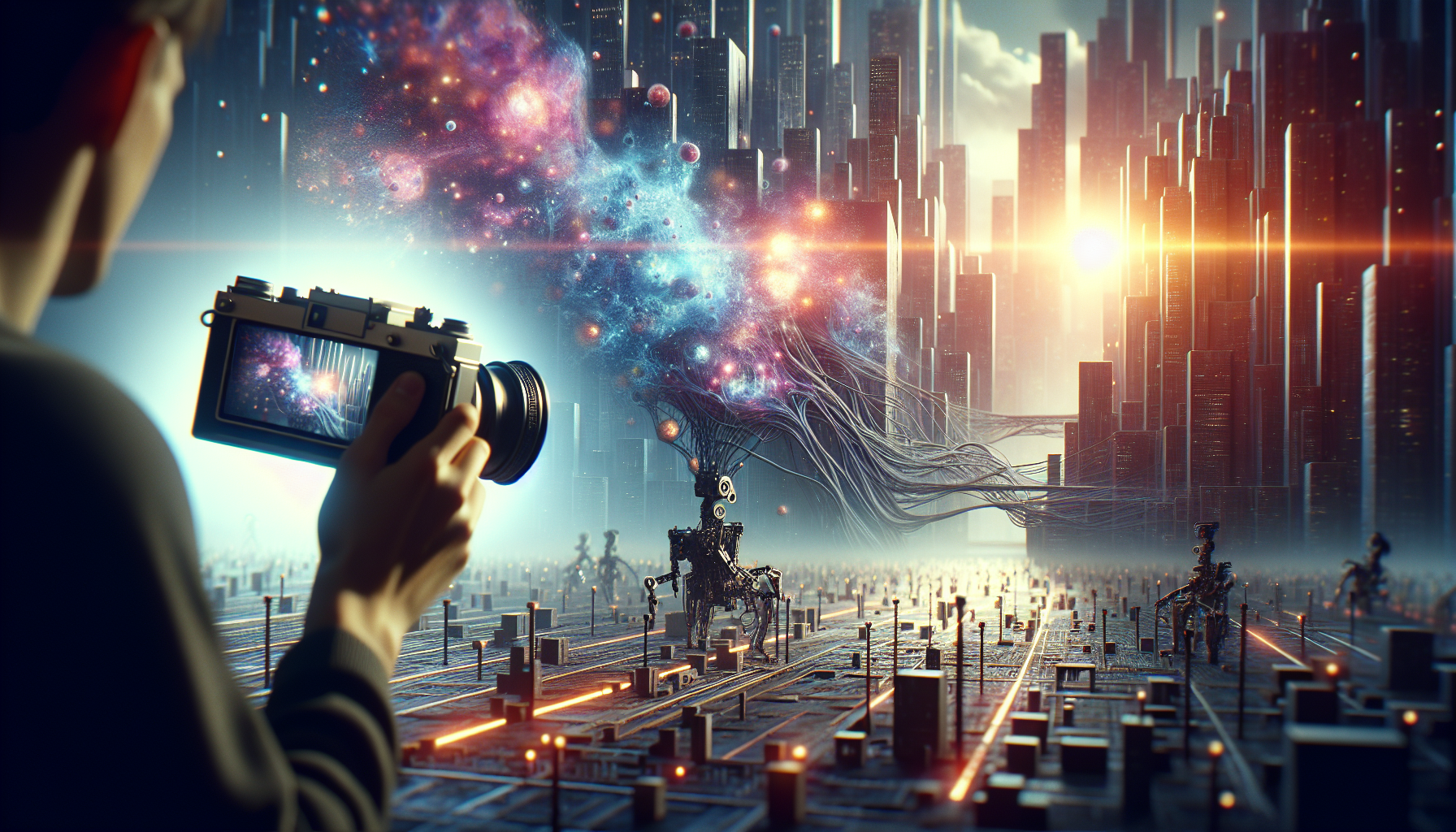
To compare our human intelligence to that of artificial intelligence, let’s return to the foundations of AI to draw a conclusion:
The theoretical foundations of AI
The theoretical foundations of AI are rooted in the field of mathematics and computer science. They arise largely from research in formal logic, algorithms and computational theory developed by figures like Alan Turing and John von Neumann. These foundations include:
- Modeling cognition: which seeks to reproduce human mental processes.
- Symbolic logic: which is based on knowledge representation and inference systems.
- Machine learning: which allows machines to learn from data and improve their performance.
- Optimization: which aims to find the best solution to a given problem in a given context.
- Game theory: which studies strategic decisions in situations of competition or cooperation.
Symbolic vs connectionist artificial intelligence
In the field of AI, there has long been a main divergence between two approaches:symbolic artificial intelligence, often associated with the manipulation of logical symbols to simulate human reasoning, and models connectionists, which take inspiration from the neuronal structure of the brain to create artificial neural networks.
These two philosophies illustrate the fundamental parallels and divergences within AI:
- Symbolic approaches are based on the explicit and formalized understanding of knowledge, while connectionist approaches rely on implicit learning patterns.
- Symbolic AI is often more transparent and its decisions more explainable, but it can be limited when faced with the complexity of certain real-world problems.
- Connectionist AI, particularly through deep learning, excels in pattern recognition and the management of large quantities of data, but sometimes suffers from a lack of transparency (black box).
Information processing: Comparison between brain and machine
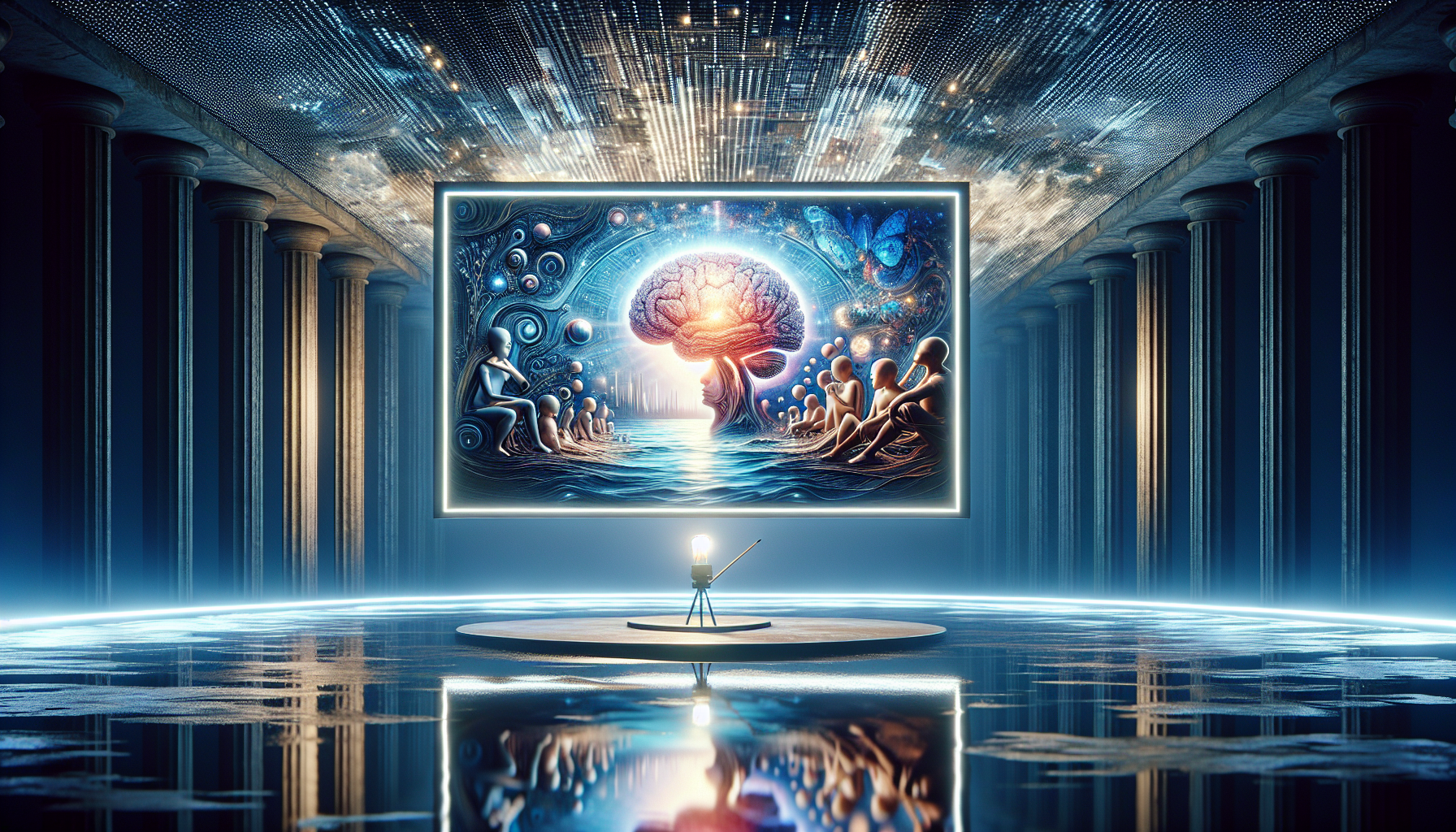
Information processing is a key function for understanding the complexity of the human brain as well as the functioning of modern computer systems. Let’s explore the similarities and differences in how human brains and machines process information.
Processing capacity and storage
The human brain has approximately 86 billion neurons, each capable of forming multiple synaptic connections, allowing it to perform complex tasks with high energy efficiency.
On the other hand, current machines, although they can handle and store huge amounts of data, still require a lot of energy to operate and lack the natural agility of the brain to learn and adapt with the same efficiency.
Learning and adaptability
The human brain is exceptional when it comes to learning and adaptability. Thanks to neuroplasticity, it can restructure itself, acquire new skills and adapt to new situations. Machines, through machine learning and artificial neural networks, are beginning to imitate this learning ability.
However, even the most advanced algorithms such as Deep Learning cannot yet match the brain’s inherent ability to understand and integrate abstract concepts organically.
Processing speed
When it comes to processing speed, machines often have the advantage. Computers can perform mathematical operations at a speed unmatched by the human brain.
However, decision-making and complex problem solving in ambiguous or changing situations are areas where the brain excels because of its ability to perform parallel tasks and make rapid heuristic judgments.
Understanding context and nuances
One of the most notable limitations of machines compared to human brains is their ability to understand context and nuance. The brain is exceptional at grasping the subtleties of language, culture, emotions, and other contextual factors, something that machines, despite advances in AI, have not yet fully mastered. AI systems like GPT-3 are taking giant steps in this direction, but there is still much to be done to achieve true contextual understanding.
In short, the comparison of information processing between brain and machine is complex and highlights the extraordinary limits and capabilities of both systems. While machines are advancing rapidly, they have not yet entirely replaced human cognitive processes in their entirety.
This coexistence between humans and machines offers immense opportunities for the future, whether in improving human capabilities or in the development of advanced AI.
Advances in machine learning: towards convergence with cognitivism?
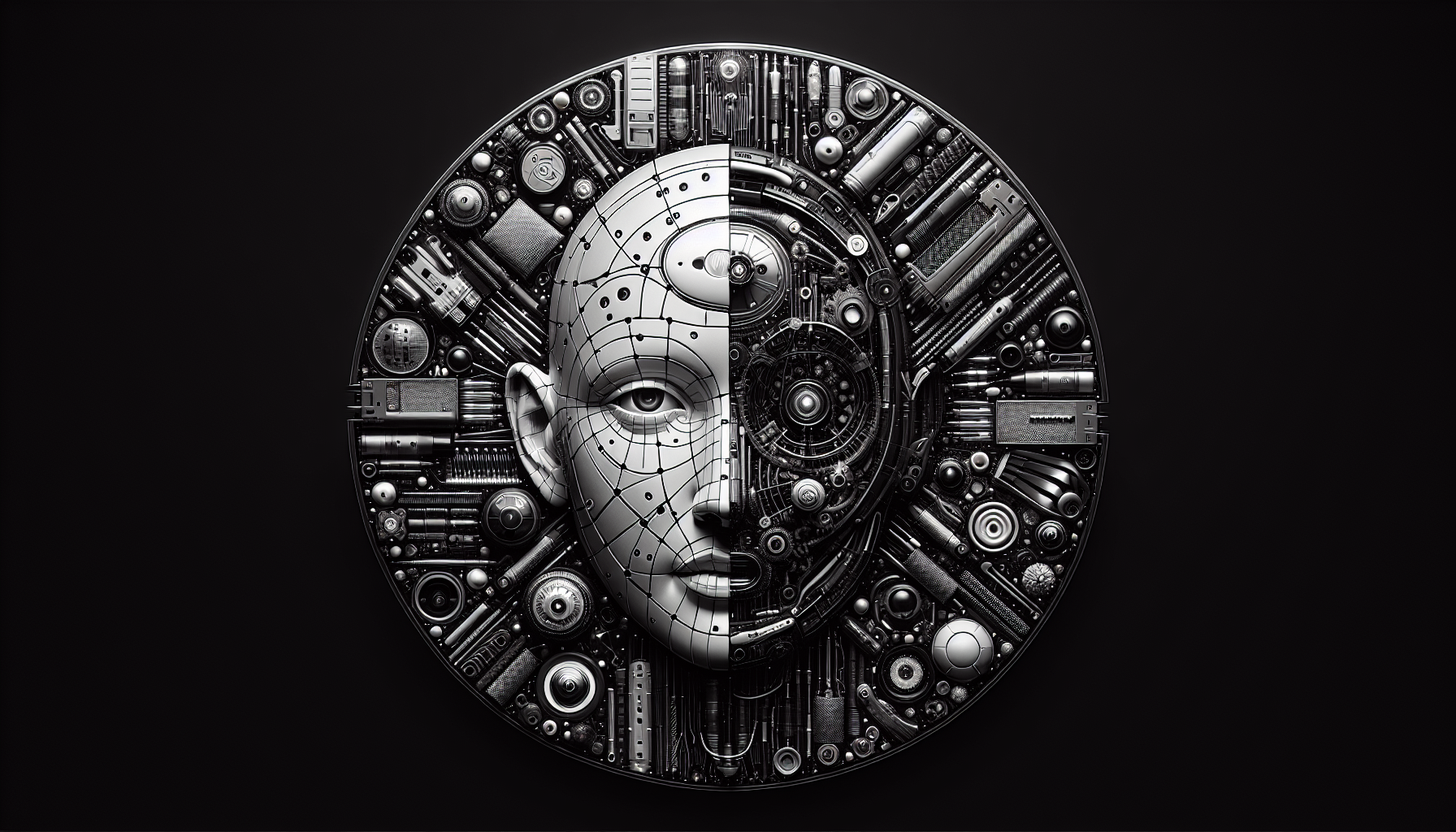
The Awakening of Cognitivism
THE cognitivism focuses on the study of thought and mental processes, seeking to understand how humans perceive, learn, remember, and solve problems. This cognitive science draws on various disciplines such as psychology, neurobiology, philosophy of mind and computer science to attempt to map the architecture and functioning of the human brain.
Parallels between machine learning and cognitivism
Many principles of machine learning find an echo in cognitivism. For example, artificial neural networks, designed to mimic the functioning of human neurons, demonstrate engineering attempts to replicate information processing as it would take place in the brain. Concepts such as supervised and unsupervised learning also reflect some human learning processes, although in a simplified way.
The contributions of machine learning to cognitivism
Innovative approaches in machine learning shed new light on the perspectives of cognitivism. By producing models that can process complex and massive datasets, machine learning offers tools for testing cognitive theories on a scale that was previously unimaginable. Additionally, developing brain-computer interfaces could revolutionize the way we understand and interact with the human brain.
Convergence between AI and cognitive sciences
There is a potential convergence between the machine learning and cognitive sciences. More specifically, computational modeling from AI can lead to better understandings of information processing in humans, and conversely, intuitions about human cognitive functioning can inspire new algorithmic architectures. Some researchers argue that this convergence could lead to forms of AI that are more robust, flexible, and capable of true intelligence.
Finally, the machine learning and cognitivism pursue parallel objectives: understanding and simulating intelligence, whether artificial or natural. Bringing these two fields together could not only accelerate the development of AI, but also deepen our understanding of the human mind. However, we are still in the early stages of this symbiotic relationship, and only the future will determine its full potential.